Multivariate imputation for missing data handling a case study on small and large data sets
Abstract
Abscent of records generally termed as missing data which should be treated properly before analysis procedes in data analysis. There were many researchers who undoubtedly mislead their research findings without proper treatment of missing data, therefore this review research try to explain the best ways of missing data handling using r programming. Generally, many researchers apply mean and median imputation but this sometimes creates bios in many situations, therefore, the researcher tries to explain some basic association among other research variables with treating missing data using r programming. The imputation process suggests five alternatives be replaced for missing data values were generated automatically and substituted easily at the process of data cleaning and data preparation. Here researcher explains two sample data for missing treatment and explains many ways for graphical interpretation of them. The first data set with 12 observation describes the easiest way of missing replacement and the second vehicle failure data from internet of 1624 records, whose missing pattern were calculated and replaced with to the respective data sets before analysis.
References
Aizaz Chaudhry, W. L. (2018). A Method for Improving Imputation and Prediction Accuracy of Highly Seasonal Univariate Data with Large Periods of Missingness. Academic Editor: Simone Morosi.
Alma, P. E.-F. (2017). © 2017 Pedersen et al. This work is published and licensed by Dove Medical Press Limited. The full terms of this license are available at https://www.dovepress. com/terms.php and incorporate the Creative Commons Attribution – Non-Commercial (unported, v3.0. Clinical Epidemiology downloaded from https://www. Dove press. com / by 128.
Buuren, S. v. (2019). Package ‘mice’December 13, 2019.
Gary King, J. H. (1998). Listwise Deletion is Evil: What to Do About Missing Data in Political Science.
Géron, A. (2017). Hands-On Machine Learning with Scikit-Learn & TensorFlow. Hands-On Machine Learning with Scikit-Learn and TensorFlow by Aurélien Géron. All rights reserved. Printed in the United States of America.
He, Y. (2010). Missing Data Analysis Using Multiple Imputation: Getting to the Heart of the Matter.
Heeringa, P. B. (2019). Multiple Imputation of Missing Data Using SAS.
Howard J, S. (2018). Experimental Design andAnalysis.
Loo, E. d. (2013). An introduction to data cleaning with R. Statistics Netherlands.
The Hague/Heerlen 2013.Reproduction is permitted, provided Statistics Netherlands is quoted as the source.
NZ, S. (2015). A guide to good survey design (4th ed). Available from. Statistics NZ Information Centre: info@stats.govt.nz.
Oehlert, G. W. (2010). A First Course InDesign and Analysis of Experiments. Design-Expert is a registered trademark of Stat-Ease, Inc.Library of Congress Cataloging-in-Publication Data.
Pasteels, J.-M. (2013). 1 Review of best practice methodologies for imputing and harmonizing data in cross-country datasets.
Roy, B. (2019). Missing Data Imputation Techniques. Data Science | Machine Learning | Deep Learning | Artificial Intelligence.
Sauro, J. (2015). 7 Ways to Handle Missing Data . W AY S T O H A N D L E M I S S I N G DATA 2, 2015.
Su, Y.-S. (2011). Multiple Imputation with Diagnostics (mi) in R.
Verzani, J. (2012). simpleR{UsingR forIntroductoryStatistics. JohnVerzani(verzani@ math. csi. cuny.edu), 2001-2.All rights reserved.
Vidhya, A. (2016). Tutorial on 5 Powerful R Packages used for imputing missing values.
Zwick, K. J. (2018). What to do when incomplete subjects information is available?Tutorial on Dealing with Missing Data.
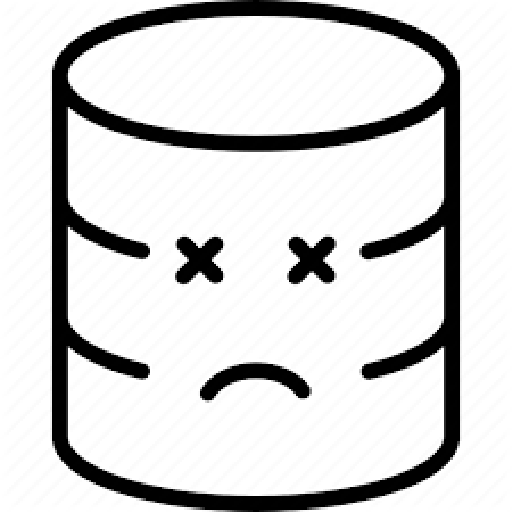